Sleep quality of professional e-Sports athletes (Counter Strike: Global Offensive)
by Victor Sanz-Milone, Paulo Yoshinori, Andrea Maculano Esteves*Email: andrea.esteves@fca.unicamp.br
Received: 19 Nov 2020 / Published: 13 Sep 2021
Abstract
Background: E-Sports athletes stay for long periods in front of televisions or monitors, with this, it is speculated that there are alterations on their sleep quality and quantity.
Aims: This study aimed to evaluate the sleep quality of professional e-Sports players, specifically the players of the Counter-Strike: Global Offense (CS: GO) game.
Methods & Results: The study was carried out with 12 young people between 17 and 25 years old. To analyze the sleep pattern, the following questionnaires were applied: Pittsburgh sleep quality index (PSQI), Morningness-eveningness Questionnaire (MEQ), Epworth sleepiness scale (EES), and Sleep Diary for 7 consecutive days. The results showed that the players were 21.58±0.5 years, most had an evening type chronotype, followed by the neither chronotype. The e-Sports athletes showed poor sleep quality, increased sleep latency, reduced sleep efficiency, and were borderline for excessive daytime sleepiness. Positive correlations have been demonstrated for: excessive daytime sleepiness, sleep latency and sleep efficiency with age, with these factors being worse in younger athletes.
Conclusion: In this context, the results suggest that CS: GO e-Sports athletes presented poor sleep quality, which were more pronounced in younger athletes.
Highlights
- Found poor sleep patterns in esports athletes as assessed in this cohort of CS:GO players
- Esports players that are older appear to have better subjective sleep quality
Introduction
The term electronic sports, also known as esports, dates back to the nineties. It started in South Korea where the preferred game type was the real-time strategy one. The rapid esports ascension in the country was aided by government measures that ensured the fast growth of Korean broadband infrastructure. With the global development of the internet, esports became more prominent, bringing to light new kinds of games, some of which are still played to this day, such as Warcraft, Starcraft, Quake, and Counter-Strike (Taylor, 2012).
There are many categories of esports. Some of the most popular are First Person Shooters (FPS), where the player has the goal to kill or avoid being killed, from his own visual point of view. Other categories include: games that simulate physical sports or workout games, involving in most times the use of interactive motion controller; Real Time Strategy, which demand quick thinking with time in between the moves; Puzzle, which are games involving logic, matching and deductive thinking; and Action Adventure, which are oriented towards combat and exploration, mostly using the third person orientation (Elliot, 2012).
In esports, players need to cope well with large 3D rendering scenarios. The athletes must display highly coordinated movements and patterns to keep controlling their characters. They also need to have a good memory and attention, two of the top qualities of a good E-athlete or esports player (Campbell, 2018). Currently, around the world, the esports have become an activity of high interest and practice among adolescents and young adults, just as traditional sports. A study by Chinese researchers demonstrated that the age range of Chinese esports athletes is between 17 to 28 years old, with the average of 19 years old, making the majority of the athletes teenagers (Meng-Lewis et al. 2020).
Studies seeking to analyze the use of electronic equipment have identified that using computers may alter sleep architecture. LeBourgeois et al. (2017) have examined the screen time and how it affects the sleep parameters of teenagers and in 90% of the cases, more screen time was associated with delayed bedtimes and shorter total sleep time in adolescents. In addition, adolescents tend to use the cellphone before and after lying down, which can negatively influence their sleep. Teenagers using cellphones before bedtime were the ones that presented with the worst sleep latency, shorter total sleep time, and had the latest sleep offset when compared with teenagers that avoided cellphones before bedtime (Amra et al. 2017).
The average sleep recommended for the young population is between 8 – 9 hours each night (Mercer, 1998), however a study in New Zealand shows that 21% of the teenagers report inadequate sleep and the number of individuals that are engaged in high levels of leisure activities (including computer and video gaming) are the ones reporting less sleep in comparison with others that are not (Dorofaeff; Denny, 2006). The literature has demonstrated that sleep has a vital role in learning and memory consolidation and it is also when the person renews attention levels (Fullagar, 2015). If someone has poor sleep quality, there might be prejudice in both memory and attention which increases the likelihood of injuries and accidents (Lowe, 2017). However, there are few studies detailing the routine and sleep profile of esports players, therefore this study seeks to identify the sleep quality of the esports athletes that play specifically the game Counter-Strike: Global Offensive (CS:GO).
Methodology
Fifteen CS:GO players between 17 and 25 years old, were invited to participate. As an inclusion criteria all the players needed to be professionals, part of the same team and have also reached level 20 of the “Gamers Club” rank and global elite rank on the CS:GO platform. Both are the most prestigious ranked classifications for this game’s community. This study was approved by the UNICAMP Research Ethics Committee (2.874.266) according to CNS Resolution No. 466/12, and to the 1995 Helsinki declaration. All participants completed informed consent.
Experimental model
The players were required the following sleep questionnaires (Pittsburgh Sleep Quality Index - PSQI, Epworth Sleepiness Scale – ESS, Morningness-eveningness Questionnaire - MEQ). The sleep diary was completed for seven consecutive days. During the period of study, the participants lived at their gaming house (Figure 1).
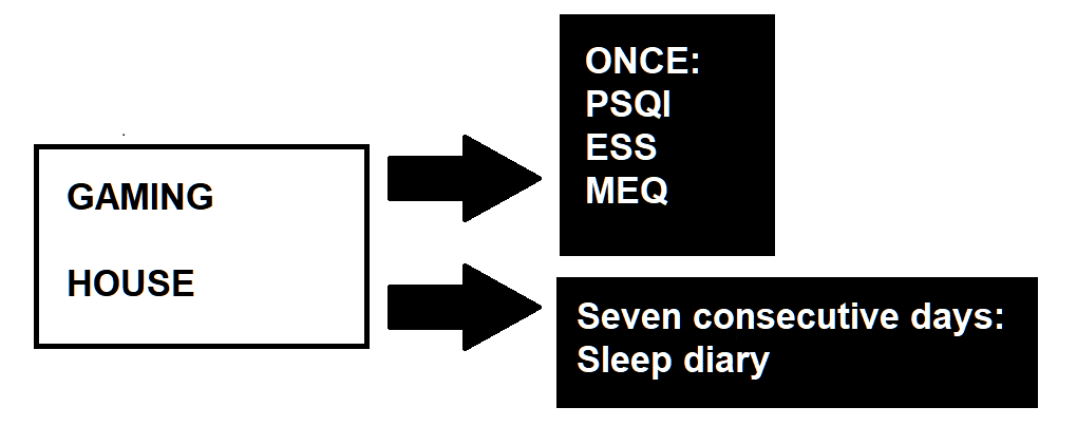
Sleep questionnaire
Morningness-eveningness Questionnaire - MEQ: The Horne and Ӧstberg’s morningness and eveningness questionnaire was used to measure the circadian preference of the athletes. It is composed of questions related to the times at which the person prefers to perform his or her daily activities, regardless of other commitments. Chronotype classification can be defined as extreme evening types (EE-types (16–30); evening types (E-types) (31–41); neither types (Ntypes) (42–58); morning types (M-types) (59–69); and extreme morning types (EM-types) (70– 86) (Horne, 1976; Benedito-Silva, 1990).
Pittsburgh Sleep Quality Index (PSQI): The PSQI was used to quantify the subjective sleep quality of all athletes in the previous month (30 days). This questionnaire has seven categories scores: subjective sleep quality; sleep latency; sleep duration; habitual sleep efficiency; sleep disturbances; use of sleeping medication; and daytime dysfunction. The sum of scores for these seven components yields one global score overall, ranging from 0–21 points. A lower PSQI score (<5) indicates higher sleep quality. On the other hand, a higher PSQI score (>5) may indicate severe difficulties in all eight components, reflecting poorer sleep quality (Buysse, 1989).
Epworth Sleepiness Scale – EES: This questionnaire allows evaluation of daytime sleepiness. It is a short and straightforward questionnaire intended to analyze sleepiness complaints concerning excessive daytime sleepiness at various moments of the individual's day. This questionnaire contains 8 questions, with a scale ranging from 0 to 3, and a global score ranging from 0 to 24 points. Scores ≥10 are considered to demonstrate excessive daytime sleepiness (Johns, 1991)[14]. The Brazilian version of the EES was used in this study (ESS-BR) as presented by Bertolazi et al. (2009).
Sleep diary: The athletes were requested to complete a sleep diary to indicate: 1) the time the person had wanted or intended to wake up; 2) the time the person woke up; 3) whether the person woke up spontaneously, by an alarm clock, or because of another (specified) disturbance; 4) the time the person got out of bed; 5) the start and end times of any daytime naps and exercises; and 6) the name, dosage, and time of any drugs used, including medication, sleep aids, caffeine, and alcohol (Mantua, 2016).
Statistical analysis
The results were expressed as mean ± standard deviation and percentage (%) for descriptive statistics. The Shapiro-Wilk test, with a 5% significance level, was used to analyse the data. The student t-test (independent) was used to analyse the quality sleep, ANOVA one way to analyse the sleep routine of the e-Sports athletes during 7 days, and the Pearson’s r was used to calculate the correlation between the age, daytime sleepiness, sleep efficiency and sleep latency. Cohen’s effect size (\(d\)) was used to determine trivial (\(d < 0.2\)), small (\(0.2 < d < 0.5\)), medium (\(0.5 < d < 0.8\)) and large (\(d > 0.8\)) effect sizes (Cohen, 1988). The critical level of significance was p < 0.05. Statistical analyses were conducted using Statistica® for Windows® (version 7.0, StatSoft Inc., Tulsa).
Results
15 esports players were initially recruited, of whom 12 completed the study as 3 decided that they did not want to answer the questionnaires or did not complete all of the questionnaires. At study entry, participants were 21±0.5 years old.
Sleep quality and chronotype
The E-types were predominant within the esports players (7), followed by N-types (5). For the PSQI, all players demonstrated a positive score for sleep complaints (PSQI> 5). In total, 75% of participants (9) had sleep efficiency below 85%. We observed a high prevalence of clinically borderline excessive daytime sleepiness (9.16±1.4). In total, 33.33% of participants were excessively sleepy during the day (ESS > 10). In addition, 58.33% had sleep latency greater than 30 minutes. All results were compared with the reference values provided for the age range (Ohayon, 2017)[18] (Table 1).
Variable | Mean (DP) |
---|---|
Chronotype | 39.58±7.0 |
Daytime Sleepiness (ESS) | 9.16±1.4 |
Pittsburgh (PSQI) | |
Sleep Latency | 38.75±7.0 |
Sleep Efficiency | 83.89±6.8 |
PSQI score | 9.25±0.9 |
Analyzing the sleep quality in conjunction with the chronotype, we noticed that the E-types players presented more alterations in the sleep variables than the N-types. The E-types also demonstrated higher sleep latency, lower sleep efficiency, higher PSQI score, with a tendency for the presence of excessive daytime sleepiness, as found below (Table 2). The effect size of Etypes on daytime sleepiness was larger, with E-types reporting a higher ESS score (d=1.36), in addition to a medium Sleep Efficiency score (d=0.70) and higher PSQI score (d=0.64).
Variable | Neither (N=5) M±SE (CI95%) |
Evening (N=7) M±SE (CI95%) |
p | Delta (%) |
Cohen’s effect size (d) |
---|---|---|---|---|---|
Sleep Latency (min) | 35.00±14.66 (-5.71-75.71) |
41.42±7.04 (24.18-58.67) | 0.67 | 18 | 0.25 |
Sleep Efficiency (%) | 93.55±14.11 (54.35-132.75) |
76.99±5.87 (62.62-91.35) | 0.25 | -18 | -0.70 |
PSQI (score) | 8.20±0.73 (6.15-10.24) |
10.00±1.51 (6.30-13.69) | 0.36 | 22 | 0.64 |
Daytime Sleepiness | 6.20±0.80 (3.97-8.42) |
11.28±2.14 (6.03-16.56) | 0.08 | 82 | 1.36 |
Sleep onset (h) | 2:26±00:59 (01:12-03:40) |
2:35±1:08 (01:31-03:38) | 0.82 | 1 | 0,14 |
Sleep offset (h) | 09:45±2:05 (07:09-12:20) |
10:03±01:51 (08:20-11:47) | 0.79 | 3 | 0.16 |
Sleep quality and age
The relation between age and sleep efficiency resulted in a Pearson correlation of r=0.82, p=0.001. There was no relation between daytime sleepiness and age (r=-0.48, p=0.10) nor a relation between sleep latency and age (r=-0.39, p=0.19). Therefore, younger athletes had lower percentages of sleep efficiency (Figure 2)
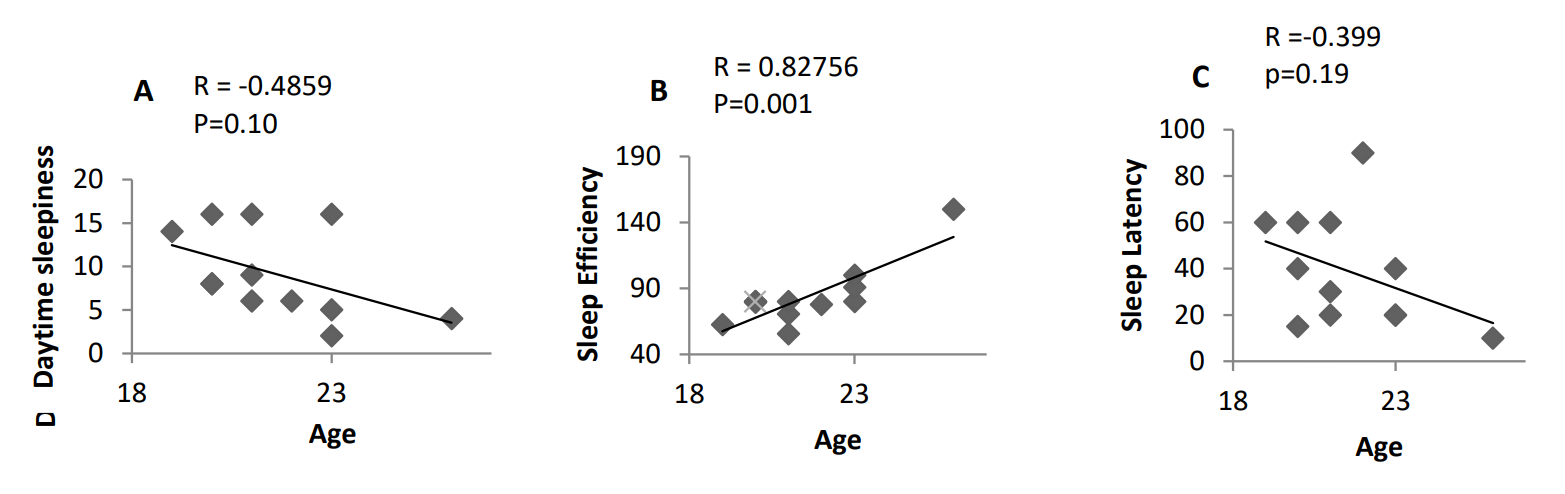
Although no statistically significant difference was found between E-types and N-types in the sleep routine, of the E-athletes of CS: GO according to the sleep diary answered for 7 consecutive days answered by the E-athletes during a normal week of training, Figure 3 demonstrates that they do not have a routine between onset and offset sleep.
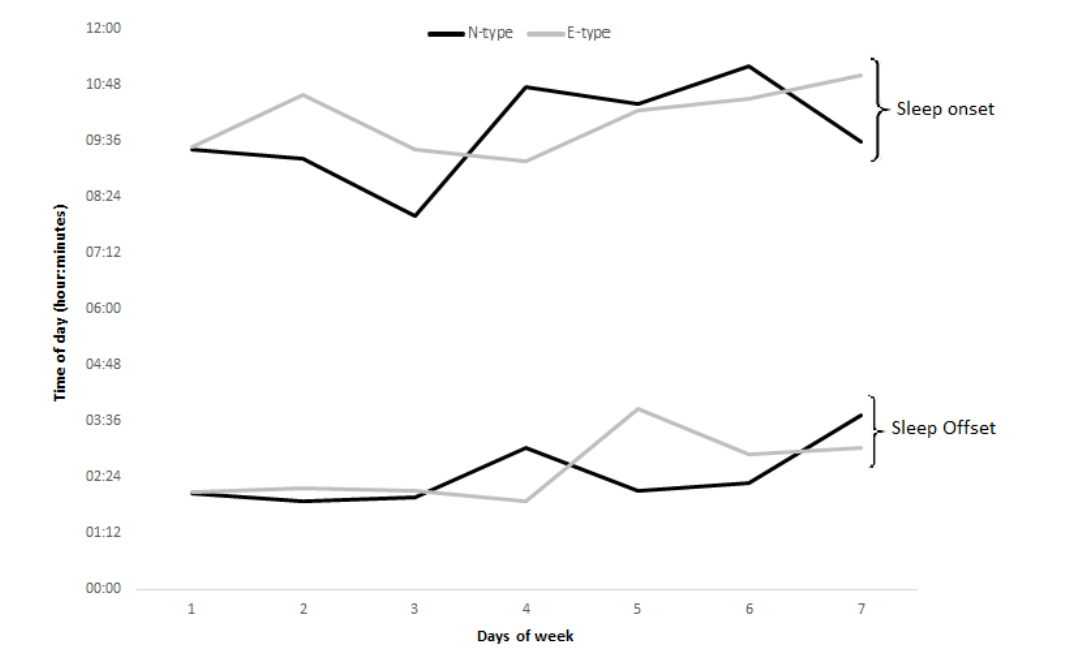
Discussion
This study described the sleep characteristics of esports players and showed this cohort to have the E-type as the predominant chronotype, as well as a poor sleep quality. E-athletes are required to have a great visual-motor and information process speed. During the game, the player must create responses to different changes in the screen made by the game and by other athletes with the use of fine motor skills and small hand muscles. This is of great importance for the athlete’s avatar to keep pushing towards victory (Bonnar, 2019). Sleep alterations can lead to sleep restriction, that in turn can cause a decrease in reaction time (Goel, 2017), in processing speed (Chee, 2015) and a slower processing of visual information (Alhola; Polo-Kantola, 2007). The reaction time can almost double if the sleep restriction persists for 7 consecutive days (Choudhary, 2016). When one or more of these three capabilities are decreased, the athlete is at a competitive disadvantage (Bonnar, 2019).
Rudolf et al. (2020) made an assessment of demographic and health behavior of video game and e-Sports players in Germany and found that the majority of participants were on their twenties and more than half were apprentice pupils or university students. Such findings correlate with this study, demonstrating that the overall age of players are equivalent in both countries. In this study, the mean age of the analyzed population was 21.4 years, same to other literature studies, showing that the E-chronotype prevails in this age range. Roenneberg et al. (2004) identified that adolescents in their early twenties have a higher tendency to the evening chronotype, which often prevails until the early stages of adult life. College students in most cases, have an irregular sleep routine, especially regarding their bedtime and wake time, with a tendency to lay down and wake up late on weekends to compensate for the sleep loss during working days (Tsai, 2004; Lund, 2010; Taylor, 2010). Without statistical difference, the esports players in this study presented with a similar sleep profile.
We can also note that the majority of the CS-GO athletes present evening chronotype, which makes them more susceptible to later bedtime and wake time. However, the routine imposed to Brazilian teenagers, with university activities or work, don’t allow them to maintain those preferred hours, making the sleep routine erratic, as seen in the sleep diary. There are a lot of factors to directly influence sleep in adolescents, such as the delay of the melatonin secretion (Kotagal, 2006), and also extrinsic factors such as the high exposure to electronic devices (Higuchi, 2005). This scenario makes them a population particularly vulnerable to sleep disturbance, in addition to sleep deprivation in most cases (Wolfson, 2003). A study made by Lee et al. (2021) investigated the sleep and wake behaviors of esports players in different countries (South Korea, Australia and USA) and identified that these players present delayed sleep patterns and more than half of the participants also reported the presence of sleep disturbances before competition. These finding are similar our own, showing that players may well present similarly regardless of the nationality.
Erratic sleep patterns can cause different impacts on adolescents and young adults, such as attention deficit (Pagel, 2007), sleepiness when driving (Cummings, 2001), depression (O’Brien, 2005), difficulty to maintain a healthy and organized social life (Carney, 2006) and also a decrease in overall health condition (Smaldone, 2007). A study made in Spain by Ortega et al. (2010) analyzed the sleep of adolescents and identified that Spanish teenagers tend to have longer sleep then teenagers from South America and other parts of the world, with an average of 8.4 hours of sleep per night. Additionally, adolescents with less tiredness were the ones more engaged in leisure time or sporting activities. In comparison to this study, we can see that an altered sleep schedule would be expected to result less sleeping time and less inclined to participate in their leisure activities. The cohort was observed to have poor sleep quality in terms of the PSQI. Also, the athletes had high sleep latency and in the case of the Etype athletes, there was also low sleep efficiency. Studies have found that watching TV is related to shorter total sleep time and worse sleep quality. Playing computer games is related to a later bedtime and shorter total sleep time (Van den Bulck, 2004; Harbard, 2016). Higuchi et al. (2005) analyzed the sleep of individuals using the computer games before sleep and demonstrated that there was an increase in sleep latency in players at bedtime. Furthermore, Ozerch et al. (2011) identified in their study that college students with later bedtime had the worst grade point average (GPA). The PSQI showed that these students also had a low subjective sleep quality. These results are similar to the ones found in this work. Araujo et al. (2013) identified that the students with the worst sleep quality were the ones currently coursing the early stages of college. These findings also corroborate with the correlations found in this study, which shows that the younger athletes are the ones with the higher sleep latency and daytime sleepiness, and lower sleep efficiency.
This study does have limitations such as the small number of participants, but the players chosen are highly ranked in competitive play. In addition, it was not possible to analyze the sleep-wake cycle using the actigraphy, although we believed an analysis like that would enhance the results found in this study. For future research, it is imperative that the population of esports players are evaluated for their sleep parameters, with different screening for different game categories. More sophisticated evaluations are also necessary, using different sleep evaluation tools such as the wristwatch actigraphy and polysomnography.
Conclusion
In this cohort of esports players of Counter-Strike: Global Offensive, we observed poor subjective sleep quality and high daytime sleepiness. Additionally, inadequate routine of sleep hygiene with fluctuant bedtime and wake time between workdays and weekends was present. The results suggest that the profile demonstrated by esports players may be prejudicial for their academic performance and also may negatively influence their gaming sessions.
References
- Alhola P, Polo-Kantola P. Sleep deprivation: Impact on cognitive performance. Neuropsychiatr Dis Treat. 2007;3:553-567.
- Amra B, Shahsavari A, Shayan-Moghadam R, Mirheli O, et al. The association of sleep and late-night cell phone use among adolescents. J Pediatr (Rio J). 2017;93:560-567.
- Araujo MFMd, Lima ACS, Alencar AMPG et al. Sleep quality assessment in college students from Fortaleza-CE. Texto & Contexto - Enfermagem. 2013;22:352-360.
- Benedito-Silva AAL, Menna-Barreto L, Marques N et al. A self-assessment questionnaire for the determination of morningness-eveningness types in Brazil. Prog Clin Biol Res. 1990;341:89-98.
- Bertolazi AN, Fagondes SC, Hoff LS et al. Portuguese-language version of the Epworth sleepiness scale: validation for use in Brazil. J Bras Pneumol. 2009;35:877-83.
- Bonnar D, Castine B, Kakoschke N, Sharp G. Sleep and performance in Eathletes: for the win! Sleep Health. 2019;5;6:647-650.
- Buysse DJ, Reynolds CF 3rd, Monk TH et al. The Pittsburgh Sleep Quality Index: a new instrument for psychiatric practice and research. Psychiatry Res. 1989;28:193-213.
- Campbell MJ, Toth AJ, Moran AP, Kowal M, Exton C. eSports: A new window on neurocognitive expertise? Prog Brain Res. 2018;240:161-174.
- Carney CE, Edinger JD, Meyer B, Lindman L, Istre T. Daily activities and sleep quality in college students. Chronobiol Int. 2006;23:623-637.
- Chee MWL. Limitations on visual information processing in the sleep-deprived brain and their underlying mechanisms. Current Opinion in Behavioral Sciences. 2015;1:56- 63.
- Choudhary AK, Kishanrao SS, Dadarao Dhanvijay AK et al. Sleep restriction may lead to disruption in physiological attention and reaction time. Sleep Sci. 2016;9:207-211.
- Cohen J. Statistical Power Analysis for the Behavioral Sciences. New York, NY: Routledge Academic. 1988
- Cummings P, Koepsell TD, Moffat JM, Rivara FP. Drowsiness, counter-measures to drowsiness, and the risk of a motor vehicle crash. Inj Prev. 2001;7:194-199.
- Dorofaeff TAD, Sleep and adolescence. Do New Zealand teenagers get enough? Journal of paediatrics and child health, 2006;42:515-520.
- Elliott L, Golub A, Ream G, Dunlap E. Video game genre as a predictor of problem use. Cyberpsychol Behav Soc Netw. 2012;15:155-161.
- Fullagar HH, Skorski S, Duffield R, Hammes D, Coutts AJ, Meyer T. Sleep and athletic performance: the effects of sleep loss on exercise performance, and physiological and cognitive responses to exercise. Sports Med. 2015;45:161-186.
- Goel N. Neurobehavioral Effects and Biomarkers of Sleep Loss in Healthy Adults. Curr Neurol Neurosci Rep. 2017;17:89.
- Harbard E, Allen NB, Trinder J, Bei B. What's Keeping Teenagers Up? Prebedtime Behaviors and Actigraphy-Assessed Sleep Over School and Vacation. J Adolesc Health. 2016;58:426-432.
- Higuchi S, Motohashi Y, Liu Y, Maeda A. Effects of playing a computer game using a bright display on presleep physiological variables, sleep latency, slow wave sleep and REM sleep. J Sleep Res. 2005;14:267-273.
- Horne JA, Ostberg OA. self-assessment questionnaire to determine morningnesseveningness in human circadian rhythms. Int J Chronobiol. 1976;4:97-110.
- Johns MW. A new method for measuring daytime sleepiness: the Epworth sleepiness scale. Sleep. 1991;14:540-545.
- Kotagal S, Pianosi P. Sleep disorders in children and adolescents. BMJ. 2006;332:828- 832.
- LeBourgeois MK, Hale L, Chang AM, Akacem LD, Montgomery-Downs HE, Buxton OM. Digital Media and Sleep in Childhood and Adolescence. Pediatrics. 2017;140:92- 96.
- Lee S, Bonnar D, Roane B et al. Sleep Ch aracteristics and Mood of Professional Esports Athletes: A Multi-National Study. Int J Environ Res Public Health. 2021; 18:664.
- Lowe CJ, Safati AX, Hall PA. The neurocognitive consequences of sleep restriction: A meta-analytic review. Neurosci Biobehav Rev. 2017;80:586-604.
- Lund HG, Reider BD, Whiting AB, Prichard JR. Sleep patterns and predictors of disturbed sleep in a large population of college students. J Adolesc Health. 2010;46:124- 132.
- Mantua J, Gravel N, Spencer RM. Reliability of Sleep Measures from Four Personal Health Monitoring Devices Compared to Research-Based Actigraphy and Polysomnography. Sensors (Basel). 2016;16:E646.
- Meng-Lewis, Wong D, Zhao Y, Lewi G. Understanding complexity and dynamics in the career development of eSports athletes. Sport Management Review, 2020:1-17.
- Mercer PW, Merritt SL, Cowell JM. Differences in reported sleep need among adolescents. J Adolesc Health, 1998;23:5:259-263.
- O'Brien EM, Mindell JA. Sleep and risk-taking behavior in adolescents. Behav Sleep Med. 2005;3:113-133.
- Ohayon M, Wickwire EM, Hirshkowitz M, et al. National Sleep Foundation's sleep quality recommendations: first report. Sleep Health. 2017;3:6-19.
- Ortega FB, Chillón P, Ruiz JR, Delgado M. et al. Sleep patterns in Spanish adolescents: associations with TV watching and leisure-time physical activity. European Journal of Applied Physiology. 2010;110;3:563-573.
- Orzech KM, Salafsky DB, Hamilton LA. The state of sleep among college students at a large public university. J Am Coll Health. 2011;59:612-619.
- Pagel JF, Forister N, Kwiatkowki C. Adolescent sleep disturbance and school performance: the confounding variable of socioeconomics. J Clin Sleep Med. 2007;3:19- 23.
- Roenneberg KT, Pramstaller PP, Ricken J, Havel M, Guth A, Merrow M. A marker for the end of adolescence. Current Biology. 2004;14:1038-1039.
- Smaldone A, Honig JC, Byrne MW. Sleepless in America: inadequate sleep and relationships to health and well-being of our nation's children. Pediatrics. 2007;119(1): 29-37.
- Rudolf K, Bickmann P, Froböse I et al. Demographics and Health Behavior of Video Game and eSports Players in Germany: The eSports Study 2019. Int J Environ Res Public Health. 2020; 17:1870.
- Taylor, TL. Raising the stakes: E-sports and the professionalization of computer gaming. Cambridge, MA:MIT Press;2012.
- Taylor DJ, Bramoweth AD. Patterns and consequences of inadequate sleep in college students: substance use and motor vehicle accidents. J Adolesc Health. 2010;46:610-612.
- Tsai LL, Li SP. Sleep patterns in college students: gender and grade differences. J Psychosom Res. 2004;56:231-237.
- Van den Bulck J. Television viewing, computer game playing, and Internet use and selfreported time to bed and time out of bed in secondary-school children. Sleep. 2004;27:101-104.
- Wolfson AR, Carskadon MA. Understanding adolescents' sleep patterns and school performance: a critical appraisal. Sleep Med Rev. 2003;7:491-506